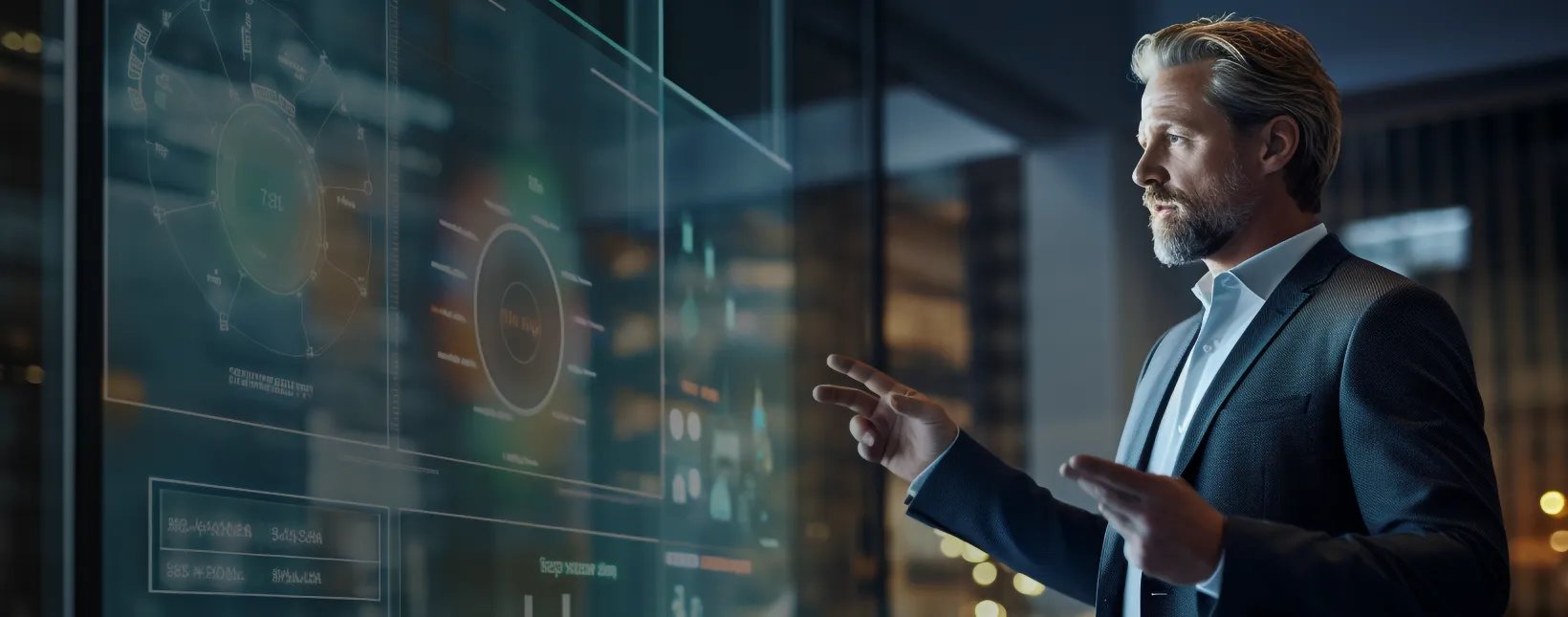
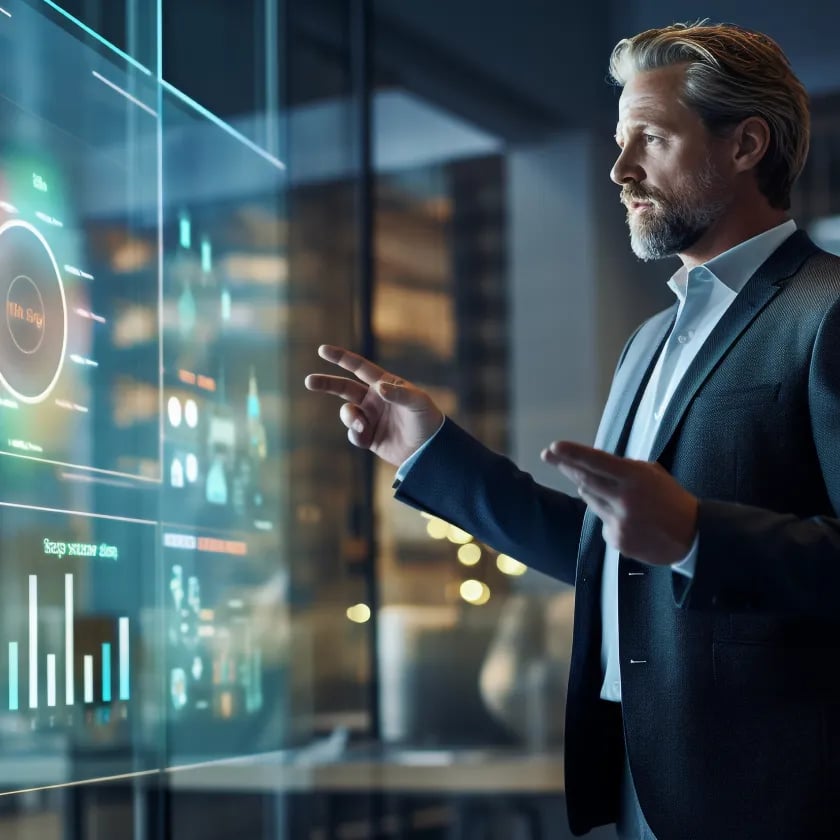
GN Demand Forecasting
ML for sales forecast
Statistical and Machine Learning algorithms for sales forecasting
GN Techonomy has realized, using Statistical and Machine Learning Algorithms, a solution for forecasting product demand – and therefore sales -, combined with Oracle Analytics for the visualization and analysis of results. Starting from taking charge of the monthly sales history detected by the ERP JD Edwards, the system performs a classification of the articles in seasonal or continuous, an analysis and a normalization of sales anomalies and spot orders per item / customer over time and, calculate the monthly forecasts by article with the possibility of splitting the monthly forecasts into weekly. This solution allows the company to plan orders in advance to promptly satisfy requests, avoid pending orders and, at the same time, limit warehouse costs.
Forecast calculation
The model created by GN Techonomy is flexible and adapts to both the series of sales history available and the life cycle of the article. The sales history is reprogrammed in the case of spot orders automatically by the system in order to avoid that a “sporadic” data could influence the forecasts. In fact, the article / customer analysis often highlights “anomalous” situations of spot sales. Therefore, before proceeding with the calculation of the forecasts on the historical sales basis, the system must analyze the spot situations and check if it is an isolated case or if the demand for that article can be considered recurring. The historian is then revised to harmonize anomalous situations in order to provide the forecasting algorithms with a historical-sales base without peak situations. There is also the possibility of managing products with a historical-sales base lower than the classic 24-month period.
As for new articles, or in any case with a sales history of less than 6 months, the model connects these articles to a series of articles of the past that may be considered similar will talk about a map of the correlations available to the User. The system analyzes the sales of the first 6 months of these goods and produces a forecast of demand for the new product.
Predictability
With each new calculation of the Forecasts, the system records a historical: the current operating forecasts are overwritten but the previous values are recorded in a historical table. For each article it is then possible to analyze how the forecasts in a given period have changed. The check has a preventive meaning when analysing the future and whether the recalculated forecasts from month to month have substantial differences: constant values indicate sales in line with the forecasts of the first period and at the same time, good reliability for the future. If the analysis, on the other hand, involves strong deviations, then the articles are put in alarm as it is necessary to analyze the critical lead times, the levels of security stock and other data of Plan. The Machine Learning system realized by GN Techonomy, analyzes both sales data and forecast trends in order to identify correlations.
Reliable forecasts - minimum 80% up to 95% are achieved thanks to the combined use of different algorithms.
A success story by GN Techonomy
Friday 19 June 2020, together with Dott. Marco Sartorelli, IT Manager of Centro Style, we discovered how Oracle Analytics and Machine Learning algorithms encoded by GN Techonomy, integrated directly with the Oracle JD Edwards ERP, allow the company to analyze, process and share detailed information, and at the same time get accurate sales forecasts.
Watch the video